The aim of this Pilot Study is to provide projections of the snow characteristics (depth, water equivalent and extension of the coverage) in different climate change scenarios, using both Global Climate Models (GCMs) and Regional Climate Models (RCMs) nested in the global models. In order to achieve this goal, two different modeling approaches have been identified:
• Direct use of the snow-related variables provided by a climate model: in this case the surface-snow module is interactive with the atmosphere and therefore the feedbacks of the snow cover changes on the climate (e. g. the snow/ice-albedo feedback) are incorporated
• Use of physical and empirical models (e. g. CH-TESSEL, UTOPIA, ETI, ACHAB-Snow, Geotop) in off-line mode, forced by the atmospheric variables produced by the climate models. In this case the effects of the feedbacks between soil and atmosphere cannot be represented but, on the other hand, it is possible to calibrate the snow models and presumably obtain a more reliable/realistic representation of the snow dynamics.
As for the first approach we have considered the most up-to-date Global Climate Models output archive, the Coupled Model Intercomparison Project Phase 5 (CMIP5), freely available on the web
http://cmip-pcmdi.llnl.gov/cmip5/. This archive provides, for each model, an ensemble of historical runs for the period 1850-2005 and the future projections for the period 2006-2100, in different climate change scenarios at global scale. Among all models, we selected those providing the variable "snow depth", thus 26 models out of the total. Their spatial resolution ranges between 0.75° and 2.8° longitude. We focused first on the Hindu-Kush Karakoram Himalaya (HKKH) region, which hosts the largest and highest mountain range in the world. This area has been subdivided into two climatically homogeneous sub-regions: 1) the Hindu-Kush-Karakoram (HKK) where precipitation is mainly due to the perturbations carried by westerly winds and occurs mainly in winter/early spring months, and 2) the Himalaya where precipitation is related to the monsoons and therefore occurs mostly between June and September.
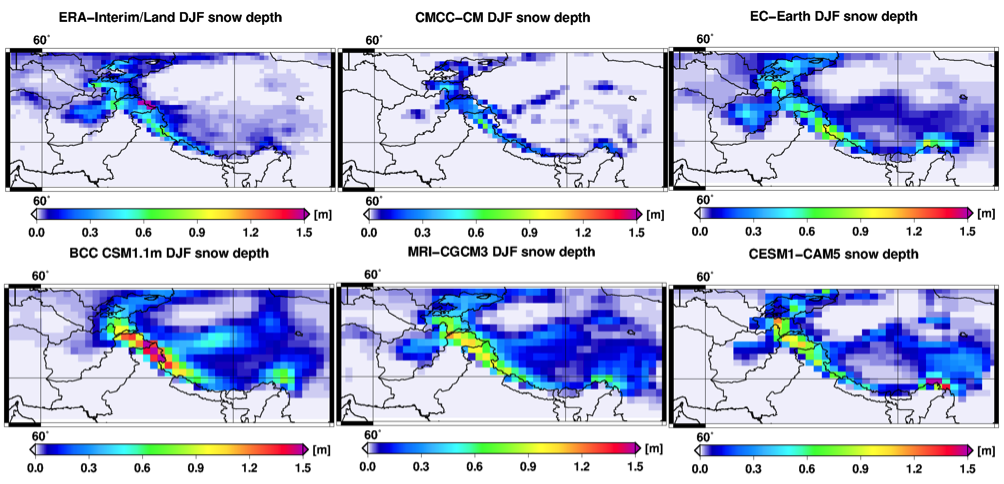
Fig 1. Average winter snow depth in the Hindu-Kush-Karakoram-Himalaya according to the high resolution (higher than 1.25° longitude) CMIP5 Global Climate Models and to the ERA-INTERIM/Land reanalysis.
First, we have examined how the Global Climate Models represent the thickness of the snowpack at monthly and seasonal scale in the HKKH region. In this area, the comparison between the models and the real characteristics of snow cover is hampered by the almost total absence of observational snow depth data. We then used the ERA-Interim/Land reanalysis and 20th Century Reanalysis as a reference for the “ground truth”. We found that the models with high spatial resolution (0.75-1.25°) are generally in better agreement with the reanalysis than those at lower spatial resolution (see Figure 1). The former show a snow depth peak on the Karakoram area and decreasing values moving towards the Himalayas and the Tibetan plateau. The analysis of the GCMs' snow depth seasonal cycle (i.e. the evolution of the snowpack thickness during the period from September to August) shows a unimodal snow depth distribution with a maximum in February/March in both HKK and Himalaya regions, and, on average, an overestimation of the snow depth compared to the ERA-Interim/Land reanalysis, as well as a non-negligible spread among the models. The future climate projections indicate a significant decrease of snow depth ranging between 8% and 28% in HKK and between 30% and 50% in the Himalayas depending on the considered scenario, RCP4.5 and RCP8.5 respectively. In the Himalayas we expect also an earlier snow melting, starting in February instead of March, with possible consequences on the seasonal distribution of melt water in downstream regions.
The second research approach aims at representing the snowpack temporal evolution by means of several physical and empirical models forced by the atmospheric variables produced by the large-scale climate models. This approach makes it possible to tune and to optimize the snow models in order to obtain more accurate performances; the drawback is that these simulations are performed in off-line mode, so the soil-atmosphere feedbacks are not represented.
Climate models provide meteorological variables at considerably lower spatial and temporal resolution compared to those registered by weather stations that are indeed generally used to validate snow models. So, as a preliminary activity, we analysed the reliability of the snow simulations depending on the "quality" and the spatial/temporal resolution of the meteorological input. In other words we have carried out simulations with different types of input data with gradually decreasing quality, and in particular we have analysed (i) the ideal case with high-quality and validated data registered in a fully instrumented weather stations, then (ii) the case of standard station data, consisting of the main meteorological variables (the other variables are estimated internally by the models' parameterizations), and finally (iii) the case of interpolated datasets with low spatial and/or temporal resolution and greater uncertainty in the measurements. The goal is to identify the models that provide the best estimates even when forced with input data at low spatial resolution (such as those provided by the GCMs).
Terzago S., von Hardenberg J., Palazzi, E., Provenzale, A.: Snowpack changes in the Hindu-Kush Karakoram Himalaya from CMIP5 Global Climate Models, accepted for publication, Journal of Hydrometeorology.